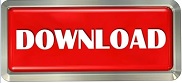

So it’s indicative of what we could have missed if we had looked only at the histogram or the fit. The higher you get, the more problematic it gets. On the right is a typical Q-Q pattern which is modeled in such a way that residual values are all found on the red line.
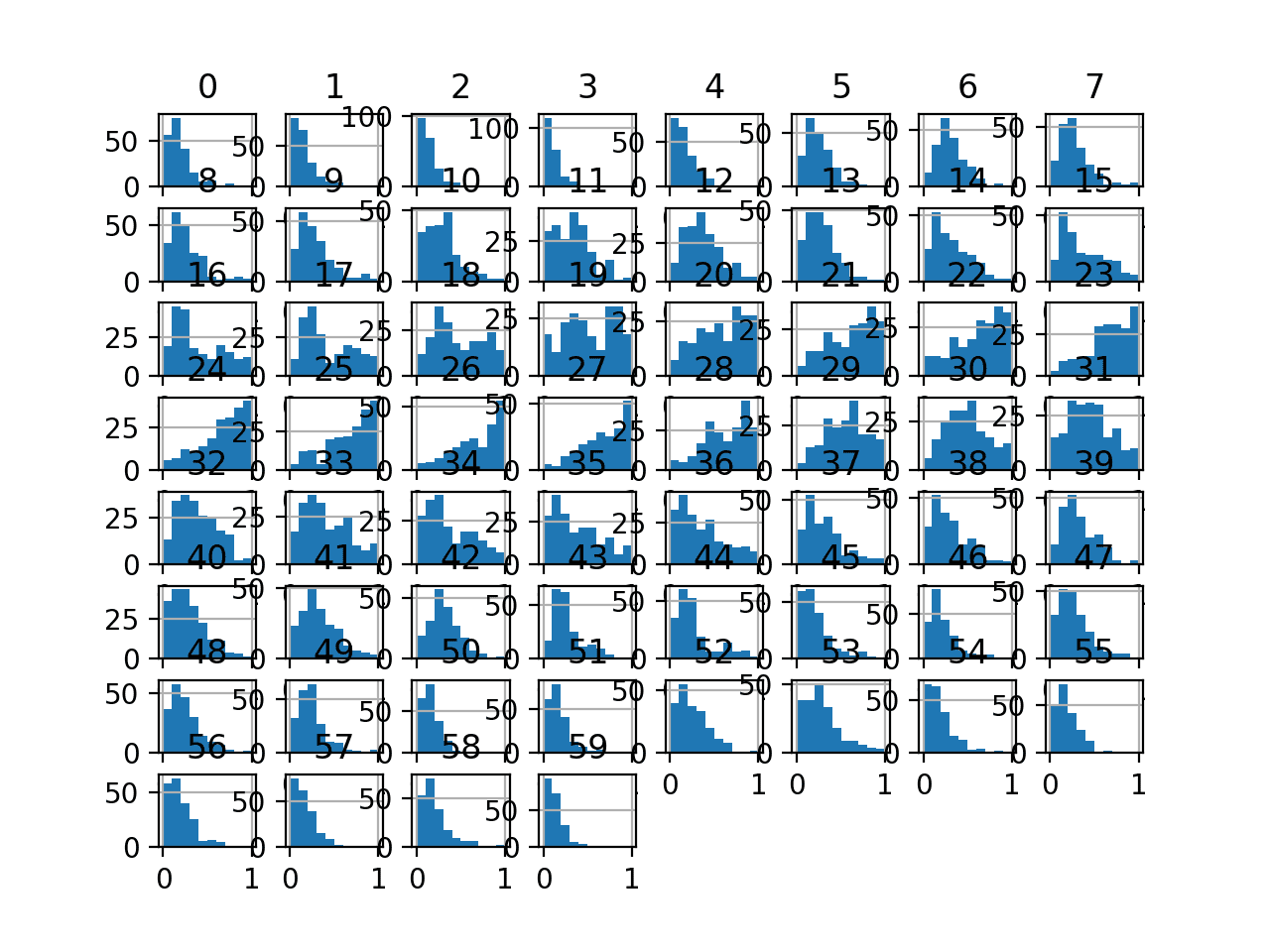
In fact, we are given more information here than in the left histogram. On the other hand, the left chart shows the disruption of this pattern. The fundamental premise behind ordinary least squares is that the residuals aren’t dependant on the function’s value, and in this model, the residuals are equally distributed. On the left is a representation of residual values versus the fitted Y values. However, the residual chart to the right looks quite evenly distributed with all the squared residuals. It just so happens that we have a lot of noise here. For our purposes, let’s say you are launching some project tomorrow and you need to come up with a workable regression line to predict model for your control program. There’s always an option to sack the statistician who accumulated data with all of the standard errors and start from scratch again. Taking all of it into consideration, you have to make your mind up as to what to do with all of these data points. Let’s suppose that there’s a good reason for a good rationale for the existence of Y, considering the red line as too high. If you didn’t know anything, imagine that the real Y was recurring at fixed intervals, amplified with X, assuming that the happening phenomena are most likely linear. First of all, this is quite an unrealistic scenario. The right graph again shows the residual values and suggested remedy to improve the situation.Ī disclaimer is needed here. On the left, we see a graph of raw data, the red line represents the ordinary least squares line, and the dashed line depicts the “actual Y” which can still be an unknown variable. We can get the following graphs via a prism of lm y x data X with uniform, periodic, and skewed noise On top of that, both model parameter estimates are hugely crucial. We can see a slight deviation of coefficients from the underlying model. Residual standard error: 4.881 on 98 degrees of freedom To make a regression can be a piece of cake. You’ve got to learn to solve the problems from daily life: to find dependency between variables ideally, to be able to differentiate signal from noise. It’s not only enough to learn two or three methods and pass an exam. To be able to handle ML and BI you need to make friends with regression equations. It’s helpful for organizing job interviews but also for solving some problems that enhance our quality in life.

If you are striving to become a data specialist, then you could go deeper and learn the ABC’s of weighted linear regression in R (the programming language and the development environment). The narrow path to machine learning (ML) leads through the rough terrain of the land of statistics.
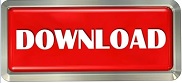